Hackers could guess your phone PIN using its
sensor data
Nanyang Technological
University
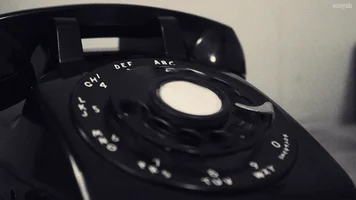
Using a combination of
information gathered from six different sensors found in smart phones and
state-of-the-art machine learning and deep learning algorithms, the researchers
succeeded in unlocking Android smart phones with a 99.5 per cent accuracy within
only three tries, when tackling a phone that had one of the 50 most common PIN
numbers.
The previous best
phone-cracking success rate was 74 per cent for the 50 most common pin numbers,
but NTU's technique can be used to guess all 10,000 possible combinations of
four-digit PINs.
Led by Dr Shivam Bhasin, NTU Senior Research Scientist at the Temasek Laboratories @ NTU, researchers used sensors in a smart phone to model which number had been pressed by its users, based on how the phone was tilted and how much light is blocked by the thumb or fingers.
The researchers
believe their work highlights a significant flaw in smart phone security, as
using the sensors within the phones require no permissions to be given by the
phone user and are openly available for all apps to access.
How the experiments
were conducted
The team of
researchers took Android phones and installed a custom application which
collected data from six sensors: accelerometer, gyroscope, magnetometer,
proximity sensor, barometer, and ambient light sensor.
"When you hold
your phone and key in the PIN, the way the phone moves when you press 1, 5, or
9, is very different. Likewise, pressing 1 with your right thumb will block
more light than if you pressed 9," explains Dr Bhasin, who spent 10 months
with his colleagues, Mr. David Berend and Dr. Bernhard Jungk, on the project.
The classification
algorithm was trained with data collected from three people, who each entered a
random set of 70 four-digit pin numbers on a phone. At the same time, it
recorded the relevant sensor reactions.
Known as deep
learning, the classification algorithm was able to give different weightings of
importance to each of the sensors, depending on how sensitive each was to
different numbers being pressed. This helps eliminate factors which it judges
to be less important and increases the success rate for PIN retrieval.
Although each
individual enters the security PIN on their phone differently, the scientists
showed that as data from more people is fed to the algorithm over time, success
rates improved.
So while a malicious
application may not be able to correctly guess a PIN immediately after
installation, using machine learning, it could collect data from thousands of
users over time from each of their phones to learn their PIN entry pattern and
then launch an attack later when the success rate is much higher.
Professor Gan Chee
Lip, Director of the Temasek Laboratories @ NTU, said this study shows how
devices with seemingly strong security can be attacked using a side-channel, as
sensor data could be diverted by malicious applications to spy on user
behaviour and help to access PIN and password information, and more.
"Along with the
potential for leaking passwords, we are concerned that access to phone sensor
information could reveal far too much about a user's behaviour. This has
significant privacy implications that both individuals and enterprises should
pay urgent attention to," said Prof Gan. Dr Bhasin said it would be
advisable for mobile operating systems to restrict access to these six sensors
in future, so that users can actively choose to give permissions only to
trusted apps that need them.
To keep mobile devices
secure, Dr Bhasin advises users to have PINs with more than four digits,
coupled with other authentication methods like one-time passwords, two-factor
authentications, and fingerprint or facial recognition.