Fake
news detector algorithm works better than a human
University of Michigan
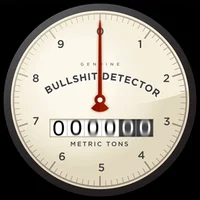
The University of
Michigan researchers who developed the system have demonstrated that it's
comparable to and sometimes better than humans at correctly identifying fake
news stories.
In a recent study, it
successfully found fakes up to 76 percent of the time, compared to a human
success rate of 70 percent. In addition, their linguistic analysis approach
could be used to identify fake news articles that are too new to be debunked by
cross-referencing their facts with other stories.
Catching fake stories
before they have real consequences can be difficult, as aggregator and social
media sites today rely heavily on human editors who often can't keep up with
the influx of news.
In addition, current debunking techniques often depend on
external verification of facts, which can be difficult with the newest stories.
Often, by the time a story is proven a fake, the damage has already been done.
Linguistic analysis
takes a different approach, analyzing quantifiable attributes like grammatical
structure, word choice, punctuation and complexity. It works faster than humans
and it can be used with a variety of different news types.
"You can imagine
any number of applications for this on the front or back end of a news or
social media site," Mihalcea said.
"It could provide users with an
estimate of the trustworthiness of individual stories or a whole news site. Or
it could be a first line of defense on the back end of a news site, flagging
suspicious stories for further review. A 76 percent success rate leaves a
fairly large margin of error, but it can still provide valuable insight when
it's used alongside humans."
Linguistic algorithms
that analyze written speech are fairly common today, Mihalcea said. The
challenge to building a fake news detector lies not in building the algorithm
itself, but in finding the right data with which to train that algorithm.
Fake news appears and
disappears quickly, which makes it difficult to collect. It also comes in many
genres, further complicating the collection process.
Satirical news, for
example, is easy to collect, but its use of irony and absurdity make it less
useful for training an algorithm to detect fake news that's meant to mislead.
Ultimately, Mihalcea's
team created its own data, crowdsourcing an online team that reverse-engineered
verified genuine news stories into fakes. This is how most actual fake news is
created, Mihalcea said, by individuals who quickly write them in return for a
monetary reward.
Study participants,
recruited with the help of Amazon Mechanical Turk, were paid to turn short,
actual news stories into similar but fake news items, mimicking the
journalistic style of the articles. At the end of the process, the research
team had a dataset of 500 real and fake news stories.
They then fed these
labeled pairs of stories to an algorithm that performed a linguistic analysis,
teaching itself distinguish between real and fake news. Finally, the team
turned the algorithms to a dataset of real and fake news pulled directly from
the web, netting the 76 percent success rate.
The details of the new
system and the dataset that the team used to build it are freely available, and
Mihalcea says they could be used by news sites or other entities to build their
own fake news detection systems.
She says that future systems could be further
honed by incorporating metadata such as the links and comments associated with
a given online news item.
A paper detailing the
system will be presented Aug. 24 at the 27th International Conference on
Computational Linguistics in Santa Fe, N.M. Mihalcea worked with U-M computer
science and engineering assistant research scientist Veronica Perez-Rosas,
psychology researcher Bennett Kleinberg at the University of Amsterdam and U-M
undergraduate student Alexandra Lefevre.
The research was
supported by U-M's Michigan Institute for Data Science and by the National
Science Foundation (grant number 1344257).