Researchers
outline how humans reduce uncertainty in social situations
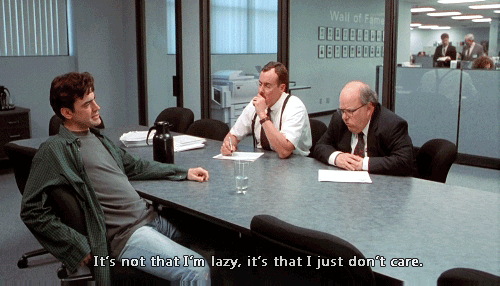
A new perspective paper from Brown
University scientists establishes a framework to apply rigorous mathematical
models of uncertainty originally developed for non-social situations, such as
whether or not to purchase a lottery ticket, to social scenarios such as
determining an interviewer’s opinion of an interviewee.
“Humans are predicting machines — our whole lives are spent trying to figure out what is the best move to do next,” said Oriel FeldmanHall, an assistant professor of cognitive, linguistic and psychological sciences at Brown.
“In general, another person’s
motivations, desires or beliefs are hidden, so we have to figure out how to
navigate through the world when we’re interacting with other people without
that knowledge. What our next action is going to be depends on how others respond.”
The framework was a collaboration
between FeldmanHall and Amitai
Shenhav, also a Brown assistant professor of cognitive, linguistic
and psychological sciences.
In the paper, they explore the
sources of social uncertainty and how people react to social uncertainty, and
they propose a model of three interrelated methods people use to reduce social
uncertainty.
The three ways people reduce social
uncertainty range from automatic, almost instinctive processes to more
cognitively demanding processes.
The authors define the first method,
automatic inference, as the process of predicting another person’s behavior
based on their appearance and the social norms of the environment.
The second method, controlled
inference, is the process of updating initial impressions using new information
such as putting yourself in the other person’s shoes and imaging how you would
behave in that situation.
Social learning, the third method,
involves updating your beliefs and actions using past experiences or secondhand
information of the person’s past behavior. People use all three processes to
different extents in order to reduce their social uncertainty.
One example presented in the paper
to illustrate the three ways people resolve social uncertainty involves lending
a stranger money. For automatic inference, one person might be willing to lend
more money to another who appears trustworthy or kind.
However, deciding to lend more money
to someone based on sharing an emotional connection or evidence of shared
values is an example of controlled inference. Social learning could then come
into play if the lender hears from a friend that the recipient is
trustworthy.
The authors also discuss the
negative consequences of mental shortcuts people use to reduce social
uncertainty.
“First impressions and stereotypes
serve a purpose as we live in very noisy worlds where we have to make snap
judgments about people all the time,” said FeldmanHall, who with Shenhav is
affiliated with Brown’s Carney Institute for Brain Science.
“You can’t get a holistic picture of
every single person you encounter on the street, but the reliance on first
impressions can go wrong when people are unwilling to seek more information and
get a bigger picture of who the person is, and instead stay narrowly focused on
their first impression.”
FeldmanHall is continuing her
research on social
uncertainty while incorporating the paper’s quantitative
framework to model a study on how people learn to trust one another in
recurrent money-lending scenarios.
She will also study how individuals
understand their social networks by tracking incoming college students as they
form their social networks and as these networks evolve over several years.
Shenhav will continue his research
modeling the neural pathways people use to make decisions, or resolve
uncertainty in non-social situations, and how they motivate themselves to
overcome cognitively demanding tasks.
In the future, Shenhav would like to
see how motivation and effort develop in children who experience early life
stress.
Both FeldmanHall and Shenhav are
supported by the National Institutes of Health’s Centers of Biomedical Research
Excellence program grant to the Center for Central Nervous System
Function (grant number P20GM12345).